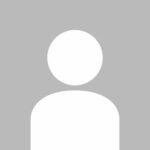
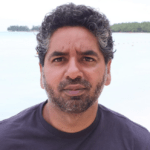
Jaysen Nelayah
Associate Professor HDR
+ 33 1 57 27 69 98
Bureau : 625B - Condorcet Building
Bio
Material scientist and transmission electron microscopy expert working on the dynamics of the structure and thermodynamics of solid-gas interfaces at the surface of metallic nanoparticles close to application conditions. Associate professor in Physics at Université Paris Cité since 2009.
with high-end scientific and technical expertise in high resolution and analytical aberration-corrected TEM, in situ and in operando environmental TEM in gas phase and development of deep-learning based methodologies for TEM images reconstruction and analysis.
Education and professional skills
2007 – 2009 Invited scientist, Stuttgart Centre for Electron Microscopy, Max Planck Institute, Germany.
2004 – 2007 Doctoral thesis in Physics, Université de Paris-Sud, Orsay, France.
1999 – 2004 Diploma, BSc and MSc in Physics, Université Paris Diderot, Paris, France.
Main research interests
Full updated list of publications on Google Scholar
1. Structure and thermodynamics of metals at the nanoscale
My contributions to these topics concern to two families of multi-component nanoparticles, bimetallic and high-entropy nanoalloys and cover their synthesis, structure and thermodynamic properties.
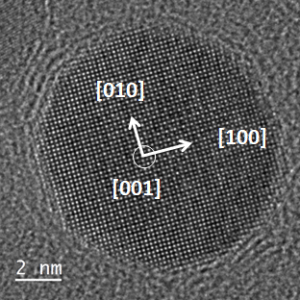
HRTEM image of a chemically-ordered L12 Au-Pd nanoparticle
Few selected contributions:
- Atomic‐Scale Insights Into the Thermal Stability of High‐Entropy Nanoalloys, S. Krouna et al., Advanced Materials, 2025
-
Direct Measurement of the Surface Energy of Bimetallic Nanoparticles: Evidence of Vegard’s Rulelike Dependence, Chmielewski et al., Phys. Rev. Lett., 2018
-
Ostwald-Driven Phase Separation in Bimetallic Nanoparticle Assemblies, Prévot et al., ACS Nano, 2016
- Long-range chemical orders in Au–Pd nanoparticles revealed by aberration-corrected electron microscopy, Nelayah et al., Nanoscale, 2014
2. In situ and operando environmental gas TEM
Since 2013, I have been involved in the implementation of in situ and operando aberration-corrected gas TEM and their integration to the study of gas-metal interactions at the surface of metallic nanoparticles down to the atomic scale.

In situ gas STEM monitoring of a gold nanoparticle under 1,3-butadiene at atmospheric pressure
Few selected contributions:
- In situ and operando transmission electron microscopy for the synthesis, fundamental understanding and catalytic applications of nanoalloys, Alloyeau et al., Advances in Physics: X, 2025
-
Atomic Scale Observation of the Structural Dynamics of Supported Gold Nanocatalysts under 1,3-Butadiene by in situ Environmental Transmission Electron Microscopy, A. Nassereddine et al., ChemCatChem, 2024
- Revealing size dependent structural transitions in supported gold nanoparticles in hydrogen at atmospheric pressure, A. Nassereddine et al., Small, 2021
3. Data engineering and machine learning-enhanced TEM
My interest lies in advancing nanomaterials investigation at the atomic scale with high-throughput by exploring novel application of machine learning approaches to TEM data reconstruction (denoising, deblurring, super resolution, …) and analysis (segmentation, classification).
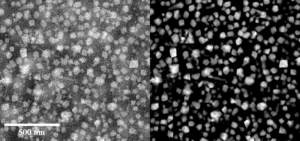
In situ liquid TEM image reconstruction using neural networks – image denoising and deblurring
Recent contributions to this topic:
- aquaDenoising: AI-enhancement of in situ liquid phase STEM video for automated quantification of nanoparticles growth, A. Moncomble et al., Ultramicroscopy, 2025
- Unlocking 3D nanoparticle shapes from 2D HRTEM images: Deep Learning for classification at atomic resolution, R. Moreau et al., in preparation, 2025
- A deep learning approach for determining the chiral indices of carbon nanotubes from high-resolution transmission electron microscopy images, D. G. Forster et al., Carbon, 2020
Teaching duties
I have been involved in teaching at UPCité since I took up my post as a lecturer in September 2009. I am attached to the Physics Department, but I also teach and have teaching responsibilities at the Denis Diderot School of Engineering (EIDD).
I teach all areas of physics (mechanics, electromagnetism, thermodynamics …) as well as Nanosciences and Materials Science, at all levels (Bachelor, Master, engineering courses and professional degrees).
At the EIDD, I’m deputy head of the Materials and Nanotechnologies specialitysince 2024. This specialisation enables students to acquire a core of skills in Physics/Chemistry, complemented by courses in nanoscience and materials science, over a three-year of theoretical and experimental training.